AI can help higher ed, but biased data can harm, warns data scientist
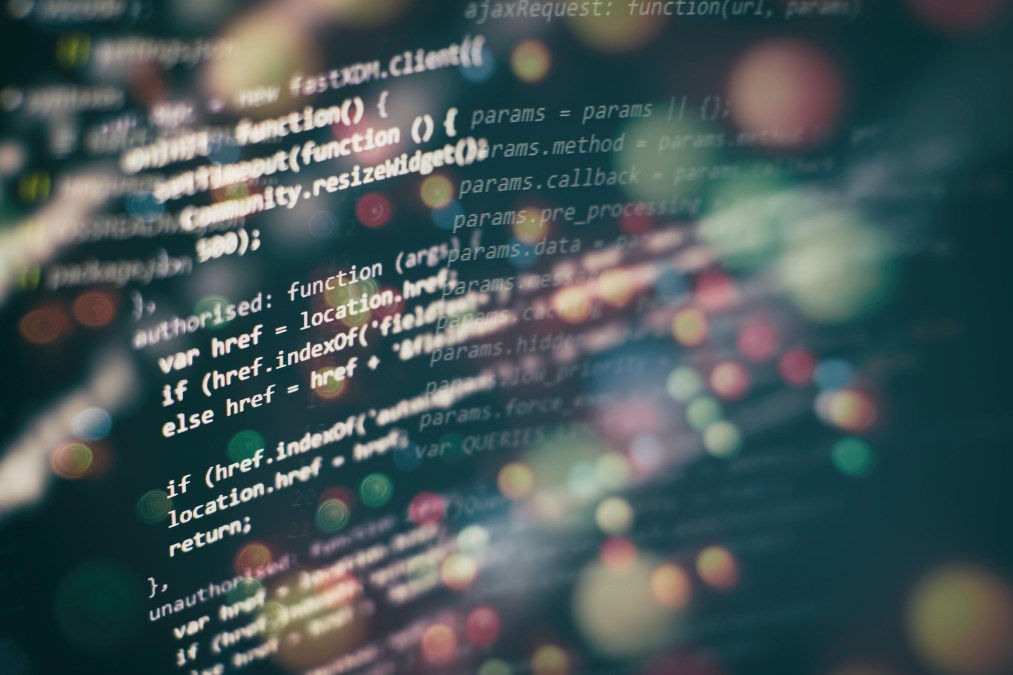
Artificial intelligence can help predict how students, faculty and higher education institutions can succeed, but understanding how AI algorithms work and how they can fail is essential for avoiding harm, data scientist Cathy O’Neil said during an online conference Thursday.
Algorithms are increasingly used in higher education with the goal to improve education and uncover the path to success for students and universities themselves. But according to O’Neil, biased data and misused algorithms can end up bringing stakeholders more harm that good.
“Algorithms are everywhere. They’re basically replacing bureaucratic processes, deciding who deserves a job, who gets insurance at what costs, who gets a loan. It even decides how long someone should go to jail based on what they think the risk of being rearrested is,” O’Neil said. “[But] no algorithm is itself a bad algorithm or a good algorithm. I can only really say the problems with algorithms in contexts.”
Mount St. Mary’s University, for example, fired several university leaders in 2016 after the university’s president used a survey tool to predict which freshman wouldn’t be successful in college and kicked them out to improve retention rates.
“The whole idea here was to get rid of these struggling freshmen before the official account day for the U.S. News and World report,” O’Neil said.
Although in the St. Mary’s case the algorithm had a harmful effect on students, using data to identify what students might be struggling with can be used to help instead of harm students, she said.
At the University of Texas, Austin, advisers used an algorithm to identify struggling students that was similar to Mount St. Mary’s. But instead of using the results to dismiss them from the university, students were connected with resources, like tutoring, to help them overcome the challenges they were facing.
In addition to how algorithms are used, what data they rely on to make predictions can also affect whether they are harmful or not, O’Neil said.
In the context of university advising, data about what a student’s interests are, their education background, socioeconomic status and where they live can be used to help them decide what to study. But the quality of the data affects the quality of the algorithm’s decision, O’Neil said.
“It’s really dangerous to take data that has embedded cultural bias and then plug it into an algorithm that memorizes and propagates this bias because it will cause bias in the future,” she said.
To address these potential failures, O’Neil said institutions need to carefully consider how algorithms can have a negative impact.
“The most important question is, for whom does this algorithm fail?,” she said. “Who will be harmed by this algorithm and what would that harm look like?”