Texas A&M predicting COVID-19 spread with deep-learning model
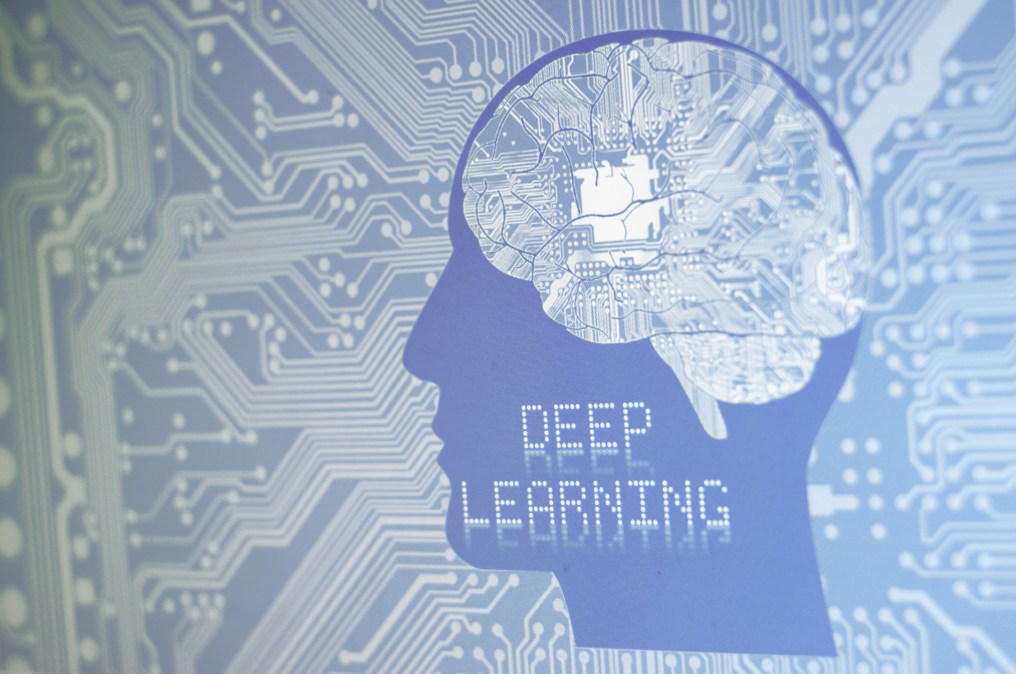
Researchers at Texas A&M University have recently begun using artificial intelligence to forecast the growth of COVID-19 cases in communities across the country.
The university is using a deep-learning model, a method of machine-learning that relies on large amounts of data, to process data related to population activities and mobility to help predict the spread of COVID-19 at a county level. Ali Mostafavi, the project’s lead researcher, said this work could help lawmakers make informed policy decisions to protect residents and mitigate spread of the virus.
“Significant opportunities exist using these big data and AI to contain the existing pandemic and also better prepare and mitigate the future pandemics,” said Mostafavi, an associate professor of civil and environmental engineering.
According to an announcement from the university last week, data fed into the model included the movement of people within communities, census data, social-distancing data, past case count growth and social demographics. The model predicted growth of future COVID-19 cases with 64% accuracy, Mostafavi said. The model’s predictions were most accurate when looking seven days ahead, with accuracy decreasing further into the future.
But being able to predict the growth of future cases is not the only important outcome of developing the model. Mostafavi said it also helps identify which factors — like population mobility or social demographics — affect the spread of the virus most, which could inform public policies, like stay-at-home orders.
“This model does not identify specific mitigation and response strategies, but it can help at different points in time to see which strategies could be effective based on various county-level features,” Mostafavi said in a university press release.
At the beginning of the pandemic, the researchers found travel-related and mobility-related factors to be important predictors of case growth, but later found other factors, such as travel to points of interests and social demographics, have become more important.
In the future, Mostafavi said he and other researchers will use new data sets to develop different types of models, including one for city-scale surveillance to predict cases at the ZIP-code level.