UCLA model predicts U.S. COVID-19 cases will peak June 1
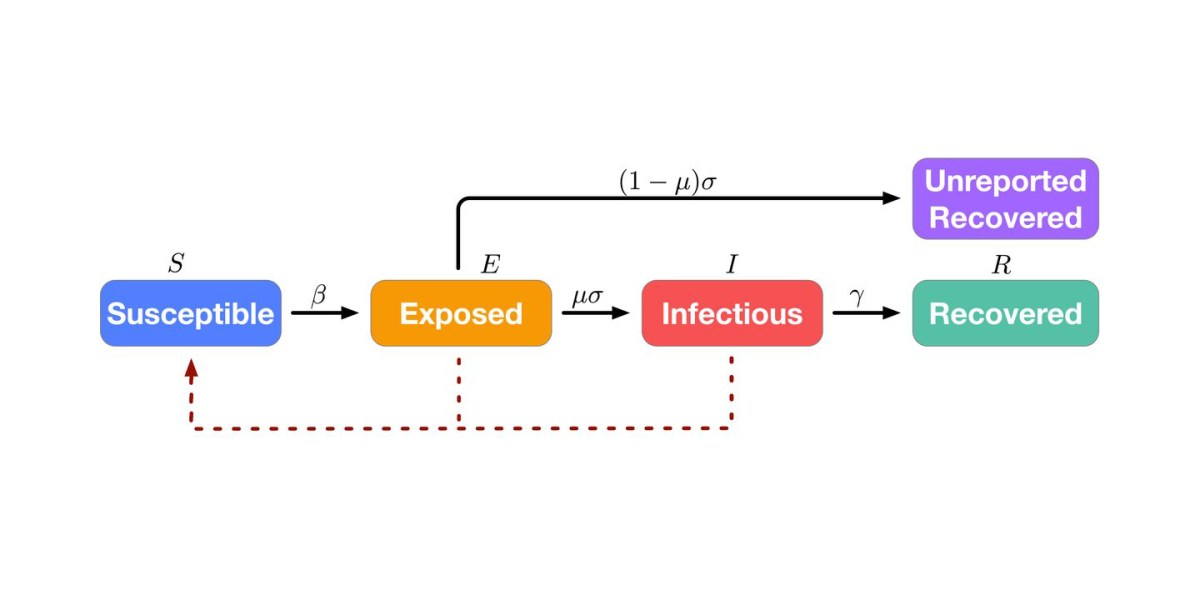
A machine-learning model developed by students at the University of California, Los Angeles is helping the Centers for Disease Control and Prevention predict the spread of the pandemic, the university announced Monday.
Rather than only using data on the number of reported cases and fatalities, UCLA’s epidemic model accounts for the various factors that affect the rate of disease spread — like the susceptibility of individuals to COVID-19 — and uses machine learning to infer the number of untested and unreported cases.
This approach allows the model to more accurately predict how quickly the disease will spread, according to Quanquan Gu, a UCLA assistant professor of computer science.
“Without any epidemic modeling, the projection by the curve-fitting model is very misleading since it only depends on the observed data pattern but ignores the underlying epidemic dynamics that drive the data,” Gu said in a press release.
The model is actually comprised of 232 sub-models — one for the U.S. overall, as well as one for each state and the 181 counties that have reported more than 1,000 confirmed cases.
So far, the model has been consistently accurate in predicting death counts in the U.S. and most states, Gu said. The tool is now one of 13 prediction models that feed into a COVID-19 Forecast Hub at the University of Massachusetts, Amherst. Data from that hub feeds into the CDC’s online forecasts to help health officials predict how the disease might continue to spread.
According to the UCLA model’s projections, the number of COVID-19 cases for the U.S. overall will peak June 1, while California will reach its peak July 1 and Los Angeles cases will peak June 7.
“Our model can help measure the effectiveness of government policies, such as social distancing, stay-at-home orders, the use of face masks and covers, or self-quarantine, as well as predicting possible resurges in cases as states reopen,” Gu said.